
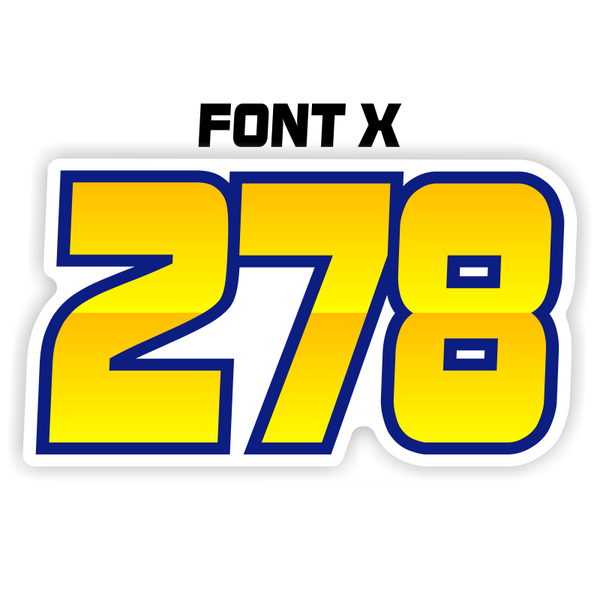
The impact of AI in histopathology is foreseen by many to be revolutionary with anticipated impact across the whole patient journey from the diagnosis of disease, assessment of prognostic and predictive features, and even stratification of patients for optimal clinical management including allocation to treatment within large clinical trials. Whilst such algorithms have the potential to support pathologists diagnostically, thus improving safety, efficiency and quality of reporting, perhaps more significant is their promise in the derivation of novel insights into disease biology and behaviour which are unachievable with a human observer. Research using digitised histopathology slides (whole slide images WSIs) for the development of artificial intelligence (AI) algorithms has increased markedly over recent years 1, 2, 3, 4, with pathology having been highlighted as being ‘ripe’ for such innovation in the UK Government’s Industrial Life Sciences Strategy 5.
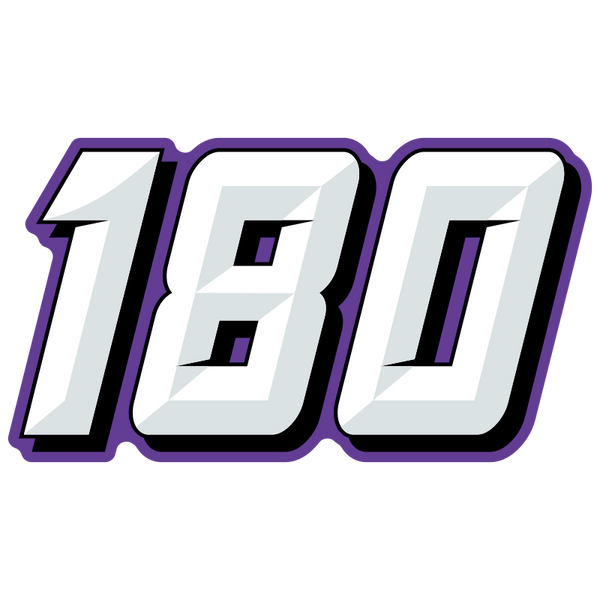
We have shown in this study that AI can be used to automate the process of quality control of large retrospective WSI cohorts to maximise their utility for research. Our model, designated as PathProfiler, indicates comparable predicted usability of images from the cohorts assessed (86–90% of WSIs predicted to be usable), and perhaps more significantly is able to predict WSIs that could benefit from an intervention such as re-scanning or re-staining for quality improvement. To demonstrate its wider potential utility, we subsequently applied our QA pipeline to the TCGA prostate cancer cohort and to a colorectal cancer cohort, for comparison. From these quality overlays the slide-level quality scores were predicted and then compared to those generated by three specialist urological pathologists, with a Pearson correlation of 0.89 for overall ‘usability’ (at a diagnostic level), and 0.87 and 0.82 for focus and H&E staining quality scores respectively. Using a two-layer approach, quality overlays of WSIs were generated from a quality assessment (QA) undertaken at patch-level at \(5\times\) magnification. In this study, we have trained and validated a multi-task deep neural network to automate the process of quality control of a large retrospective cohort of prostate cases from which glass slides have been scanned several years after production, to determine both the usability of the images at the diagnostic level (considered in this study to be the minimal standard for research) and the common image artefacts present. focus, or use traditional machine-learning methods, which are unable to classify the range of potential image artefacts that should be considered.
#DIRT 5 IMAGE QUALITY OR PERFORMANCE MANUAL#
However, manual quality control of large cohorts of WSIs by visual assessment is unfeasible, and whilst quality control AI algorithms exist, these focus on bespoke aspects of image quality, e.g.

Such resources, therefore, become very important, with the need to ensure that their quality is of the standard necessary for downstream AI development. Glass slides from retrospective cohorts, some with patient follow-up data are digitised for the development and validation of artificial intelligence (AI) tools. Research using whole slide images (WSIs) of histopathology slides has increased exponentially over recent years.
